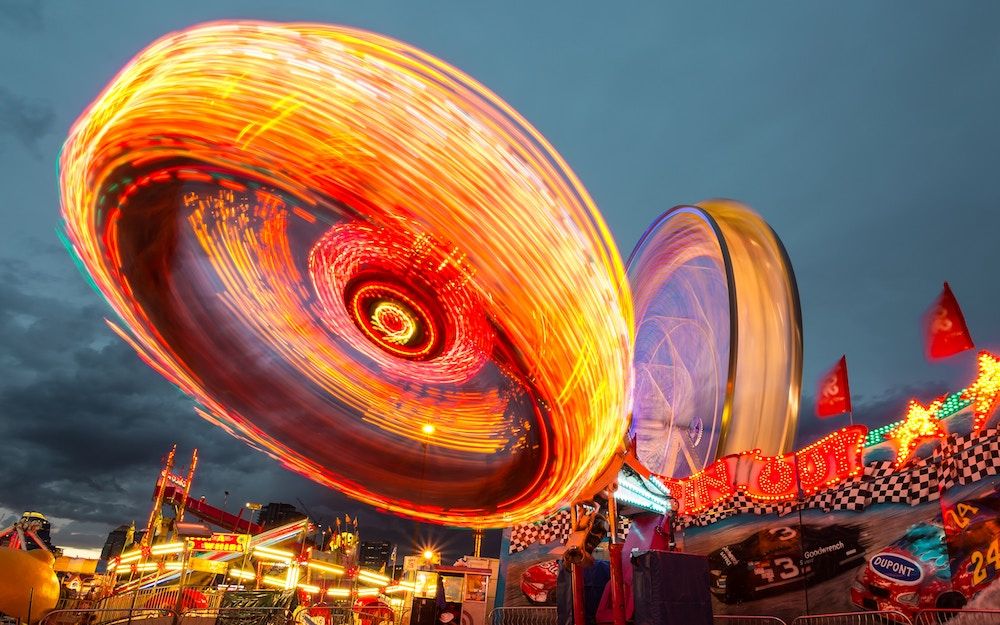
In the past articles of our series “AI for Small and Medium Businesses” you learned why AI should be on every small-business owner’s mind and how small and medium businesses can use AI to automate core processes such as marketing, HR, finance, operations and product development.
In this article we’ll teach you how to get started with AI if you are a small or medium business. We will introduce you to a process that can easily be applied to any company no matter its size. You will also learn that you likely already possess most of the capabilities needed for implementing AI in your organization.
Why?
In a recent Harvard Business Review article calledAI for the Real World, Professor Thomas H. Davenport highlighted the importance of “looking at AI through the lens of business capabilities rather than technologies”. That’s exactly the core of the methodology metriq promotes: to understand, challenge and reimagine your organization’s processes and then use artificial intelligence to enhance them.
It’s important to highlight that processes don’t occur in a vacuum.The transformation or addition of value – the main goal of most business processes – traditionally comes from the people executing a series of tasks that form a given process. This is where artificial intelligence plays a crucial role.
More than a roadmap for automation, we want to introduce you to a practical roadmap for developing human-robot collaborations that enhance both human and machine capabilities. You don’t have to be a tech giant using state-of-the-art AI technology to start implementing artificial intelligence.
Technology actually represents only ⅓ of the process, with the other ⅔ represented by process and data mapping (both associated with very low technical capabilities).
Re-imaging a process
To start, you have to understand what you want to or can automate. In this phase, it’s crucial to identify pain points and frustrations that slow down processes or demotivate employees. It’s equally important to estimate the ROI of automating an entire workflow or individual tasks.
Process Maps
As a small business owner, you probably have a deep understanding of the main processes that underlie your company. However, as happens in many small businesses, that knowledge may be kept in your head or in departmental silos across your organization.
The first objective for re-imagining processes is to map out the existing ones in order to bring transparency, assign responsibility and account operational variations and risks . In this phase you will be define the objectives of your process and the tasks or micro-tasks (the smallest actions required by a stakeholder) that make the process move forward.
Objectives are the result of tasks or usually a set of tasks that result in a final outcome. A detailed process map will allow you to not only have a thorough understanding of the goal of the process, but also to start measuring its performance.
Workflow participants
The first phase of your process mapping consists of identifying the stakeholders or workflow participants. In this step you will need to conduct functional interviews about the process, the individuals you believe are involved and the tasks they accomplish. In exchanging with these stakeholders, you should discover how tasks are done, how long each task takes and why, what emotional rapport is felt relative to a task and the assumptions of the stakeholder about how better to accomplish it.
Your goal is to identify pain points and frustrations (the level of negative emotion attached to a task and the time constraints or frictions with tools, systems or other stakeholders).
Process maps
Once you have interviewed all the stakeholders related to a process, and have mapped out the different stages of a user’s journey within a process, it’s time to distill that process into a workflow with stakeholders – known in the design world as an Experience Map.
Experience Maps depict how a user interacts with a process and how she experiences it functionally, emotionally and mentally. Everything the user does, feels, and thinks at each stage must be detailed and linked to the objective and tasks which amount to interactions. Your goal here is to obtain a precise view of each touchpoint and begin to develop an understanding of the frustrations engendered by low-value, manual work in order to remove or reduce them. The example below represents an experience map for a product (Snapchat), but could as well be used for a process.
Digitizing a process
The last step of the process mapping phase is taking offline tasks and putting them online. In this phase you’ll use APIs and analytics tools to capture the data generated throughout a process and analyze it. Your ultimate goal is to bring transparency to the stakeholders and evaluate the performance of the process.
More than analytical outputs, your KPIs become data inputs that influence how you optimize your business processes. The data collected through a digital medium will serve as the basis for calculating the possible ROI of an automation.
Data maps
As seen, objectives are the results of tasks. Let’s put this in the context of a physical object for a moment. A piece of wood becomes a chair through a combination of tasks that transform its initial state. One could imagine designing the chair, cutting the wood or painting the chair as a few of the many tasks that would be necessary. The piece of wood “flows” through each step of the process, and in each step humans or machines execute tasks that transform or add value to the wood, ultimately allowing the designer to achieve her goal of creating a chair.
But what happens when a process is based on information? Using the chair example, in order to order the appropriate quantity of wood for her line of chairs, our designer will need to know how many units of each line were sold over the last 12 months. She asks the sales department to send her a report. In order to compile a report, her colleagues have to gather a bunch of data from different sources and consolidate it. In this case, we’re operating in the context of data flows.
So what is a data flow? A data flow is the movement of specific pieces of information between or across tools, services, algorithms and software. It allows a task to be executed and an objective to be attained functionally (or digitally). In order for each data flow to occur they must be managed by manual or automated tasks or micro-tasks. As an example, the micro-tasks are interactions such as searching for data points, selecting relevant information, copying information into a visualization tool and sending out the report to the designer. All of these interactions require data flows in order to move the process along.
Processes contain several data flows. Therefore, in order to prepare your automations, the last step that ties together interactions with data is to map out these data flows for each task. In a Data Map you will be analyzing the flows of data from sources to destinations (as inputs and outputs), their availability (if they are already actionable or still need to be identified and made available).
Launching automations
Once you have mapped out the pain points, the possible ROI of the automations and the processes to be automated, it’s time to launch the automations. To develop your automations, you can either trust the task to your internal teams (if they are trained in AI and automation) or hire external providers.
Another advantage is that as you have mapped out the ROI of the automations in the previous phases, you will have a clear view of how much you can invest in automations and how much return these investments will generate.
No matter which option you choose, it’s important to understand that the investment you make here will eventually be accounted for over the preceding months and largely depends on the volume and value of the tasks you are automating. Calculating this horizon is ultimately what determines your willingness to invest and your expectations regarding cost savings.
We know, it might still sound like an overwhelming task to get your organization automated, that’s why we have three simple rules to make the process simpler and less risky:
Rule 1: Start small, iterate and grow
Automating many processes or even tasks at the same time can be extremely complex. The ideal approach is to launch pilots. From this starting point, you will be able to iterate towards the automation of more tasks or expand existing automations through AI. You can always evaluate the ROI or increase in ROI of each automation or expansion of automation. A Harvard Business School study of 152 automation projects revealed that highly ambitious moon shots are less likely to be successful than “low-hanging fruit” projects that enhance business processes.
Rule 2: Be dumb, before being smart.
As we’ve seen in a previous article, there are two types of automation, Robotic Process Automation (or RPA) and Intelligent Process Automation (or IPA).
- RPA is a “dumber” form of automation. In robotic process automation, the software robots are configured to execute steps identically to a human user. They are configured (or “trained”) using demonstrative steps, rather than being programmed using code-based instructions. Broadly speaking, a software robot is a virtual worker who can be rapidly “trained” by a manager in a way that is similar to how an employee would train a colleague. Robots have an additional advantage that they learn much faster and retain knowledge better than humans.
- IPA, goes further, and adds intelligence to RPA. Like a RPA, an IPA mimics the tasks carried out by humans, and over time and with the use of machine learning, learns to perform them even better, autonomously.
Just like in rule number one, avoid being too ambitious. Forgo moonshots involving the addition of intelligence to your processes straight away. Instead, favor putting in place “dumb automations” in order to test new process configurations and start collecting data about digital and automated tasks.
Once you feel you have stabilized the process and are sure you have chosen the right tasks to be automated, go ahead and add the machine learning algorithms that will enable the tasks to become intelligent.
Think about that sales report our chair designer requested. The sales teams could use robots to search and select relevant information, copy that information into a report and send it out to the designer. All the movement of data between the various tools, services and software could be done with RPAs. Our designer would have much more precise information much faster. She would only need to analyze the reports to decide to buy more or less oak or teak for the next lines of chairs.
Imagine now the designer suspects that the sales are heavily impacted not only by type of wood, but also by weather patterns in the last 6 months and current color trends in home-decoration magazines. She would take these three elements into consideration when making a decision, but normally this process would be done manually and take several weeks. To start with, she would have to trust her memory about the weather in the last 6 months, then she would start analyzing the sales reports and reading countless home-decoration magazines. An overwhelming task!
By using IPA we could make that task much simpler. By crossing weather data, with past sales and images from home-decoration magazines, we would be able to create a model that would indicate to the designer with a certain level of confidence the types of wood she should be buying. That algorithm would be fed every month with new data and improve autonomously. The designer would only need to decide whether to trust this recommendation system or not. Her work would become much more focused on creativity than on data crunching.
Rule 3: Adopt a bottom-up approach
A recent study from MIT shows that artificial Intelligence will replace tasks, not jobs. “Tasks within jobs typically show considerable variability in ‘suitability for machine learning’ while few (if any) jobs can be fully automated using machine learning. Machine learning technology can transform many jobs in the economy, but full automation will be less significant than the re-engineering of processes and the reorganization of tasks.”
As a leader, it’s your role to reduce ambiguity and make clear to your employees that the ultimate goal of your automations is building human-robot collaborations and not replacing humans by robots. Your employees shouldn’t be threatened by machines.Rather, they should be comfortable in passing repetitive low-value tasks to robots, so they can focus on more creative and strategic ones.
To accomplish this, your organization must adopt a bottom-up approach of letting your employees choose which micro-tasks should be automated. Once you’ve figured out what processes are more prone to automation in terms of ROI and organizational impact, start involving the people responsible for the tasks in the project that will be soon automated. Make sure they understand that by automating mundane, repetitive tasks, they can make their work much more pleasant and impactful.
Even if machines can perform certain tasks much more accurately and faster than humans, at the end of the day, there are still many things that humans do much better than machines. We have immense creative abilities that allow us to extend the boundaries of what is possible. Furthermore, we’re social beings who can develop a sense of humor, have empathy, be self-critical, think about the future and decide to change. To sum up, in this new world of automated organizations, humans and robots shouldn’t be seen as competing against each other, but rather as extensions of each other.